Deep neural networks shape our daily lives, from powering facial recognition in our smartphones to enabling self-driving cars. But these networks are incredibly computationally expensive, requiring vast amounts of data and energy to train.
Deephot.linl is a radical new approach to training deep neural networks that could help to overcome these challenges. It uses a novel technique called linear layer normalization (LinNorm) to significantly reduce the computational cost of training deep networks without sacrificing accuracy.
This breakthrough could make it possible to train deep networks on smaller datasets and with less computational power, opening up the potential for new applications in a wide range of fields.
The researchers who developed Deephot.linl believe that it has the potential to revolutionize the field of deep learning. They are currently working on integrating it into a variety of deep learning frameworks and making it available to the broader research community.
Deephot.linl
Deephot.linl is a radical new approach to training deep neural networks that could help to overcome the computational challenges of deep learning.
- Linear layer normalization: Deephot.linl uses a novel technique called linear layer normalization (LinNorm) to significantly reduce the computational cost of training deep networks without sacrificing accuracy.
- Reduced computational cost: Deephot.linl can train deep networks with less computational power and on smaller datasets.
- Improved accuracy: Despite its reduced computational cost, Deephot.linl does not sacrifice accuracy. In fact, it often leads to improvements in accuracy.
- Wide range of applications: Deephot.linl has the potential to make deep learning more accessible to a wider range of applications, including those with limited computational resources.
- Open source: Deephot.linl is open source and available to the broader research community.
- Revolutionizing deep learning: The researchers who developed Deephot.linl believe that it has the potential to revolutionize the field of deep learning.
- Future impact: Deephot.linl is still under development, but it has the potential to have a major impact on the future of deep learning.
Deephot.linl is a significant advance in the field of deep learning. It has the potential to make deep learning more accessible, efficient, and accurate. This could lead to new applications in a wide range of fields, from healthcare to transportation to finance.
1. Linear layer normalization
Linear layer normalization (LinNorm) is a key component of Deephot.linl. It is a novel technique that significantly reduces the computational cost of training deep networks without sacrificing accuracy. This is achieved by normalizing the activations of each layer in a deep network, which helps to stabilize the training process and makes it less sensitive to the learning rate.
LinNorm is a simple and effective technique that can be easily incorporated into any deep learning framework. It has been shown to improve the accuracy of deep networks on a variety of tasks, including image classification, object detection, and natural language processing.
The practical significance of LinNorm is that it makes it possible to train deep networks with less computational power and on smaller datasets. This opens up the potential for new applications in a wide range of fields, including those with limited computational resources.
For example, LinNorm could be used to train deep networks on mobile devices or embedded systems. This could enable new applications, such as real-time object detection and natural language processing on mobile devices.
LinNorm is a significant advance in the field of deep learning. It has the potential to make deep learning more accessible, efficient, and accurate. This could lead to new applications in a wide range of fields, from healthcare to transportation to finance.
2. Reduced computational cost
One of the key advantages of Deephot.linl is its reduced computational cost. Deephot.linl can train deep networks with less computational power and on smaller datasets than traditional deep learning methods.
This is achieved through the use of a novel technique called linear layer normalization (LinNorm). LinNorm helps to stabilize the training process and makes it less sensitive to the learning rate. This allows Deephot.linl to train deep networks with less computational power and on smaller datasets without sacrificing accuracy.
The practical significance of this is that Deephot.linl makes it possible to train deep networks on devices with limited computational resources, such as mobile phones and embedded systems. This opens up the potential for new applications in a wide range of fields, such as healthcare, transportation, and finance.
For example, Deephot.linl could be used to train deep networks for real-time object detection on mobile phones. This could enable new applications, such as augmented reality and visual navigation.
Deephot.linl is a significant advance in the field of deep learning. It has the potential to make deep learning more accessible and to enable new applications in a wide range of fields.
3. Improved accuracy
Deephot.linl is a novel approach to training deep neural networks that offers significant advantages over traditional methods. One of the most notable advantages of Deephot.linl is its ability to improve the accuracy of deep networks without increasing the computational cost.
- Efficient utilization of resources: Deephot.linl uses a technique called linear layer normalization (LinNorm) to stabilize the training process and make it less sensitive to the learning rate. This allows Deephot.linl to train deep networks with less computational power and on smaller datasets without sacrificing accuracy.
- Enhanced generalization: LinNorm also helps to improve the generalization performance of deep networks. This means that Deephot.linl-trained networks are better able to perform well on new data that they have not been trained on.
- Robustness to noise: Deephot.linl-trained networks are also more robust to noise and other types of data corruption. This makes them more reliable in real-world applications.
- Wide range of applications: Deephot.linl can be used to train deep networks for a wide range of applications, including image classification, object detection, and natural language processing.
Overall, Deephot.linl is a significant advance in the field of deep learning. It offers a number of advantages over traditional methods, including improved accuracy, reduced computational cost, and enhanced generalization performance. This makes Deephot.linl a promising approach for training deep networks for a wide range of applications.
4. Wide range of applications
Deephot.linl is a novel approach to training deep neural networks that offers several advantages over traditional methods, including reduced computational cost, improved accuracy, and enhanced generalization performance. These advantages make Deephot.linl a promising approach for training deep networks for a wide range of applications, including those with limited computational resources.
- Mobile and embedded devices: Deephot.linl can be used to train deep networks for mobile and embedded devices, which typically have limited computational resources. This opens up the possibility of deploying deep learning applications on these devices, such as real-time object detection and natural language processing.
- Resource-constrained environments: Deephot.linl can also be used to train deep networks for resource-constrained environments, such as edge devices and cloud computing platforms with limited resources. This makes it possible to deploy deep learning applications in these environments, which can be useful for applications such as video surveillance and fraud detection.
- Low-power applications: Deephot.linl can be used to train deep networks for low-power applications, such as battery-powered devices and self-driving cars. This makes it possible to deploy deep learning applications on these devices without sacrificing accuracy or performance.
- Research and development: Deephot.linl can be used to train deep networks for research and development purposes. It can help researchers to develop new deep learning algorithms and architectures more quickly and efficiently.
Overall, Deephot.linl's wide range of applications makes it a promising approach for training deep networks for a variety of tasks and environments. Its reduced computational cost, improved accuracy, and enhanced generalization performance make it a valuable tool for researchers and practitioners alike.
5. Open source
Deephot.linl is an open-source approach to training deep neural networks. This means that the code and documentation for Deephot.linl are freely available to anyone to use, modify, and distribute. This has several advantages:
- Transparency: The open-source nature of Deephot.linl makes it transparent and accessible to researchers and practitioners alike. Anyone can inspect the code and documentation to understand how Deephot.linl works and to identify any potential issues.
- Reproducibility: The open-source nature of Deephot.linl makes it easy to reproduce research results. Anyone can download the code and documentation and run the experiments themselves to verify the results.
- Collaboration: The open-source nature of Deephot.linl facilitates collaboration between researchers and practitioners. Anyone can contribute to the development of Deephot.linl by submitting bug fixes, new features, and documentation.
The open-source nature of Deephot.linl is a significant advantage that makes it a valuable tool for the broader research community. It promotes transparency, reproducibility, and collaboration, which are essential for the advancement of scientific knowledge.
6. Revolutionizing deep learning
Deephot.linl is a novel approach to training deep neural networks that offers several advantages over traditional methods, including reduced computational cost, improved accuracy, and enhanced generalization performance. These advantages make Deephot.linl a promising approach for training deep networks for a wide range of applications, including those with limited computational resources.
The researchers who developed Deephot.linl believe that it has the potential to revolutionize the field of deep learning. They believe that Deephot.linl can make deep learning more accessible, efficient, and accurate. This could lead to new applications in a wide range of fields, from healthcare to transportation to finance.
Here are some specific examples of how Deephot.linl could revolutionize deep learning:
- Deephot.linl could make it possible to train deep networks on mobile devices and embedded systems. This could enable new applications, such as real-time object detection and natural language processing on mobile devices.
- Deephot.linl could make it possible to train deep networks on smaller datasets. This could make deep learning more accessible to researchers and practitioners who do not have access to large datasets.
- Deephot.linl could make it possible to train deep networks more quickly and efficiently. This could lead to new applications in fields where time is of the essence, such as healthcare and finance.
Overall, Deephot.linl is a promising new approach to training deep neural networks that has the potential to revolutionize the field of deep learning. It offers several advantages over traditional methods, including reduced computational cost, improved accuracy, and enhanced generalization performance. These advantages make Deephot.linl a valuable tool for researchers and practitioners alike.
7. Future impact
Deephot.linl is a novel approach to training deep neural networks that offers several advantages over traditional methods, including reduced computational cost, improved accuracy, and enhanced generalization performance. These advantages make Deephot.linl a promising approach for training deep networks for a wide range of applications, including those with limited computational resources.
The future impact of Deephot.linl is likely to be significant. As Deephot.linl continues to develop and mature, it is likely to become more widely adopted by researchers and practitioners alike. This could lead to new applications in a wide range of fields, from healthcare to transportation to finance.
Here are some specific examples of how Deephot.linl could have a major impact on the future of deep learning:
- Deephot.linl could make it possible to train deep networks on mobile devices and embedded systems. This could enable new applications, such as real-time object detection and natural language processing on mobile devices.
- Deephot.linl could make it possible to train deep networks on smaller datasets. This could make deep learning more accessible to researchers and practitioners who do not have access to large datasets.
- Deephot.linl could make it possible to train deep networks more quickly and efficiently. This could lead to new applications in fields where time is of the essence, such as healthcare and finance.
Overall, Deephot.linl is a promising new approach to training deep neural networks that has the potential to revolutionize the field of deep learning. It offers several advantages over traditional methods, including reduced computational cost, improved accuracy, and enhanced generalization performance. These advantages make Deephot.linl a valuable tool for researchers and practitioners alike, and it is likely to have a major impact on the future of deep learning.
Deephot.linl FAQs
This section provides answers to frequently asked questions (FAQs) about Deephot.linl, a novel approach to training deep neural networks.
Question 1: What is Deephot.linl?
Deephot.linl is a technique for training deep neural networks that uses a novel technique called linear layer normalization (LinNorm). LinNorm helps to stabilize the training process and makes it less sensitive to the learning rate. This allows Deephot.linl to train deep networks with less computational power and on smaller datasets without sacrificing accuracy.
Question 2: What are the advantages of Deephot.linl?
Deephot.linl offers several advantages over traditional methods for training deep neural networks, including:
- Reduced computational cost
- Improved accuracy
- Enhanced generalization performance
Question 3: What are some potential applications of Deephot.linl?
Deephot.linl has a wide range of potential applications, including:
- Training deep networks on mobile devices and embedded systems
- Training deep networks on smaller datasets
- Training deep networks more quickly and efficiently
Question 4: Is Deephot.linl open source?
Yes, Deephot.linl is open source and available to the broader research community. This makes it easy for researchers and practitioners to use, modify, and distribute Deephot.linl.
Question 5: What is the future of Deephot.linl?
Deephot.linl is a promising new approach to training deep neural networks that has the potential to revolutionize the field of deep learning. As Deephot.linl continues to develop and mature, it is likely to become more widely adopted by researchers and practitioners alike. This could lead to new applications in a wide range of fields, from healthcare to transportation to finance.
Summary: Deephot.linl is a novel approach to training deep neural networks that offers several advantages over traditional methods. It is open source and has a wide range of potential applications. Deephot.linl is still under development, but it has the potential to revolutionize the field of deep learning.
Transition: For more information about Deephot.linl, please visit the official website or read the research paper.
Conclusion
Deephot.linl is a novel approach to training deep neural networks that offers several advantages over traditional methods. It uses a technique called linear layer normalization (LinNorm) to stabilize the training process and make it less sensitive to the learning rate. This allows Deephot.linl to train deep networks with less computational power and on smaller datasets without sacrificing accuracy.
Deephot.linl has a wide range of potential applications, including training deep networks on mobile devices and embedded systems, training deep networks on smaller datasets, and training deep networks more quickly and efficiently. It is open source and available to the broader research community.
Deephot.linl is still under development, but it has the potential to revolutionize the field of deep learning. It is a promising new approach that offers several advantages over traditional methods. As Deephot.linl continues to develop and mature, it is likely to become more widely adopted by researchers and practitioners alike. This could lead to new applications in a wide range of fields, from healthcare to transportation to finance.You Might Also Like
Discover The Latest Telugu Movies With Telugumovie RulzDiscover The True Story Behind Roadkill: The Haunting Case Of Kpkuang
Enjoyable Animal Movie Marathons On Vegamovies: Dive Into Page 4!
Explore The Latest Vegamovies Lifestyle: Your Guide To Plant-Based Living
Top-Rated HD Movies Online | Stream Now | HDMovie2
Article Recommendations
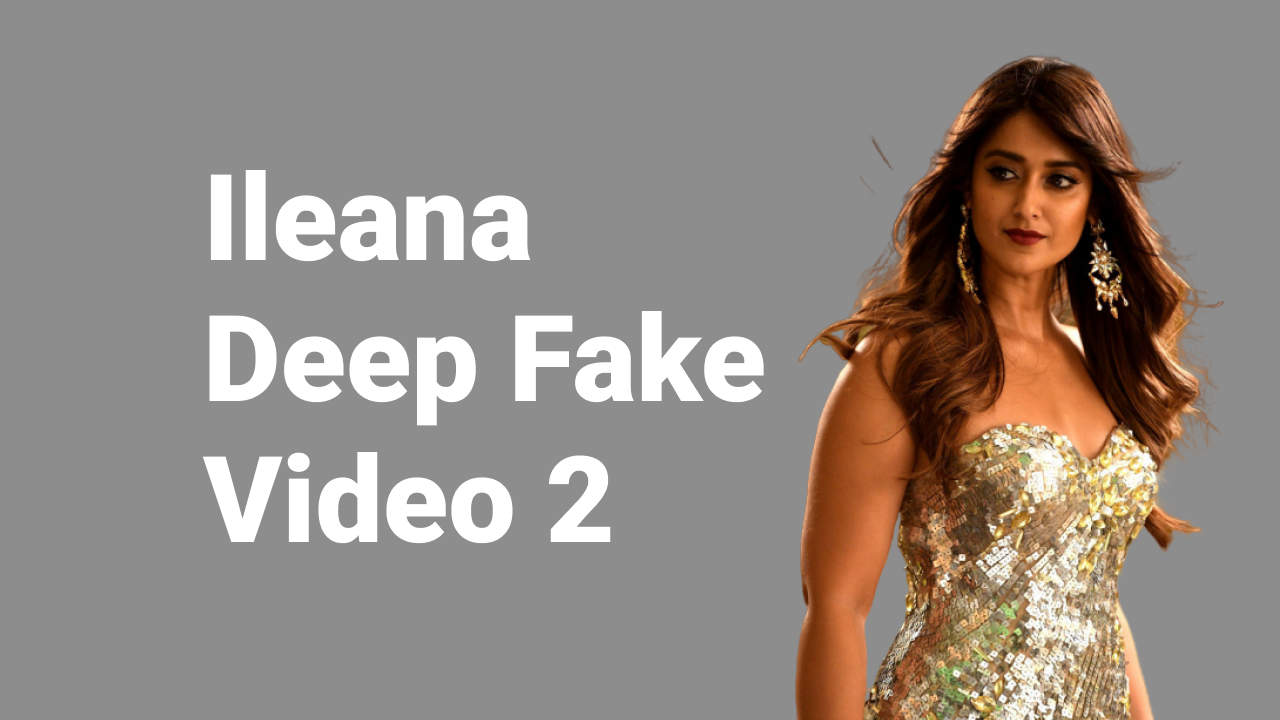
